Google Cloud CMIP6 Public Data: Basic Python Example
Overview
This notebooks shows how to query the Google Cloud CMIP6 catalog and load the data using Python.
Prerequisites
Concepts |
Importance |
Notes |
---|---|---|
Necessary |
||
Helpful |
Familiarity with metadata structure |
Time to learn: 10 minutes
Imports
from matplotlib import pyplot as plt
import numpy as np
import pandas as pd
import xarray as xr
import zarr
import fsspec
import nc_time_axis
%matplotlib inline
plt.rcParams['figure.figsize'] = 12, 6
Browse Catalog
The data catatalog is stored as a CSV file. Here we read it with Pandas.
df = pd.read_csv('https://storage.googleapis.com/cmip6/cmip6-zarr-consolidated-stores.csv')
df.head()
activity_id | institution_id | source_id | experiment_id | member_id | table_id | variable_id | grid_label | zstore | dcpp_init_year | version | |
---|---|---|---|---|---|---|---|---|---|---|---|
0 | HighResMIP | CMCC | CMCC-CM2-HR4 | highresSST-present | r1i1p1f1 | Amon | ps | gn | gs://cmip6/CMIP6/HighResMIP/CMCC/CMCC-CM2-HR4/... | NaN | 20170706 |
1 | HighResMIP | CMCC | CMCC-CM2-HR4 | highresSST-present | r1i1p1f1 | Amon | rsds | gn | gs://cmip6/CMIP6/HighResMIP/CMCC/CMCC-CM2-HR4/... | NaN | 20170706 |
2 | HighResMIP | CMCC | CMCC-CM2-HR4 | highresSST-present | r1i1p1f1 | Amon | rlus | gn | gs://cmip6/CMIP6/HighResMIP/CMCC/CMCC-CM2-HR4/... | NaN | 20170706 |
3 | HighResMIP | CMCC | CMCC-CM2-HR4 | highresSST-present | r1i1p1f1 | Amon | rlds | gn | gs://cmip6/CMIP6/HighResMIP/CMCC/CMCC-CM2-HR4/... | NaN | 20170706 |
4 | HighResMIP | CMCC | CMCC-CM2-HR4 | highresSST-present | r1i1p1f1 | Amon | psl | gn | gs://cmip6/CMIP6/HighResMIP/CMCC/CMCC-CM2-HR4/... | NaN | 20170706 |
The columns of the dataframe correspond to the CMI6 controlled vocabulary.
Here we filter the data to find monthly surface air temperature for historical experiments.
df_ta = df.query("activity_id=='CMIP' & table_id == 'Amon' & variable_id == 'tas' & experiment_id == 'historical'")
df_ta
activity_id | institution_id | source_id | experiment_id | member_id | table_id | variable_id | grid_label | zstore | dcpp_init_year | version | |
---|---|---|---|---|---|---|---|---|---|---|---|
973 | CMIP | NOAA-GFDL | GFDL-ESM4 | historical | r3i1p1f1 | Amon | tas | gr1 | gs://cmip6/CMIP6/CMIP/NOAA-GFDL/GFDL-ESM4/hist... | NaN | 20180701 |
1766 | CMIP | NOAA-GFDL | GFDL-ESM4 | historical | r2i1p1f1 | Amon | tas | gr1 | gs://cmip6/CMIP6/CMIP/NOAA-GFDL/GFDL-ESM4/hist... | NaN | 20180701 |
8074 | CMIP | NOAA-GFDL | GFDL-CM4 | historical | r1i1p1f1 | Amon | tas | gr1 | gs://cmip6/CMIP6/CMIP/NOAA-GFDL/GFDL-CM4/histo... | NaN | 20180701 |
22185 | CMIP | IPSL | IPSL-CM6A-LR | historical | r8i1p1f1 | Amon | tas | gr | gs://cmip6/CMIP6/CMIP/IPSL/IPSL-CM6A-LR/histor... | NaN | 20180803 |
22298 | CMIP | IPSL | IPSL-CM6A-LR | historical | r2i1p1f1 | Amon | tas | gr | gs://cmip6/CMIP6/CMIP/IPSL/IPSL-CM6A-LR/histor... | NaN | 20180803 |
... | ... | ... | ... | ... | ... | ... | ... | ... | ... | ... | ... |
522952 | CMIP | MRI | MRI-ESM2-0 | historical | r7i1p1f1 | Amon | tas | gn | gs://cmip6/CMIP6/CMIP/MRI/MRI-ESM2-0/historica... | NaN | 20210813 |
523274 | CMIP | MRI | MRI-ESM2-0 | historical | r6i1p1f1 | Amon | tas | gn | gs://cmip6/CMIP6/CMIP/MRI/MRI-ESM2-0/historica... | NaN | 20210907 |
523712 | CMIP | CMCC | CMCC-CM2-SR5 | historical | r3i1p2f1 | Amon | tas | gn | gs://cmip6/CMIP6/CMIP/CMCC/CMCC-CM2-SR5/histor... | NaN | 20211108 |
523721 | CMIP | CMCC | CMCC-CM2-SR5 | historical | r2i1p2f1 | Amon | tas | gn | gs://cmip6/CMIP6/CMIP/CMCC/CMCC-CM2-SR5/histor... | NaN | 20211109 |
523769 | CMIP | EC-Earth-Consortium | EC-Earth3-Veg | historical | r1i1p1f1 | Amon | tas | gr | gs://cmip6/CMIP6/CMIP/EC-Earth-Consortium/EC-E... | NaN | 20211207 |
635 rows × 11 columns
Now we do further filtering to find just the models from NCAR.
df_ta_ncar = df_ta.query('institution_id == "NCAR"')
df_ta_ncar
activity_id | institution_id | source_id | experiment_id | member_id | table_id | variable_id | grid_label | zstore | dcpp_init_year | version | |
---|---|---|---|---|---|---|---|---|---|---|---|
56049 | CMIP | NCAR | CESM2-WACCM | historical | r2i1p1f1 | Amon | tas | gn | gs://cmip6/CMIP6/CMIP/NCAR/CESM2-WACCM/histori... | NaN | 20190227 |
56143 | CMIP | NCAR | CESM2-WACCM | historical | r3i1p1f1 | Amon | tas | gn | gs://cmip6/CMIP6/CMIP/NCAR/CESM2-WACCM/histori... | NaN | 20190227 |
56326 | CMIP | NCAR | CESM2-WACCM | historical | r1i1p1f1 | Amon | tas | gn | gs://cmip6/CMIP6/CMIP/NCAR/CESM2-WACCM/histori... | NaN | 20190227 |
59875 | CMIP | NCAR | CESM2 | historical | r1i1p1f1 | Amon | tas | gn | gs://cmip6/CMIP6/CMIP/NCAR/CESM2/historical/r1... | NaN | 20190308 |
61655 | CMIP | NCAR | CESM2 | historical | r4i1p1f1 | Amon | tas | gn | gs://cmip6/CMIP6/CMIP/NCAR/CESM2/historical/r4... | NaN | 20190308 |
61862 | CMIP | NCAR | CESM2 | historical | r5i1p1f1 | Amon | tas | gn | gs://cmip6/CMIP6/CMIP/NCAR/CESM2/historical/r5... | NaN | 20190308 |
62691 | CMIP | NCAR | CESM2 | historical | r2i1p1f1 | Amon | tas | gn | gs://cmip6/CMIP6/CMIP/NCAR/CESM2/historical/r2... | NaN | 20190308 |
63131 | CMIP | NCAR | CESM2 | historical | r3i1p1f1 | Amon | tas | gn | gs://cmip6/CMIP6/CMIP/NCAR/CESM2/historical/r3... | NaN | 20190308 |
63266 | CMIP | NCAR | CESM2 | historical | r6i1p1f1 | Amon | tas | gn | gs://cmip6/CMIP6/CMIP/NCAR/CESM2/historical/r6... | NaN | 20190308 |
64615 | CMIP | NCAR | CESM2 | historical | r8i1p1f1 | Amon | tas | gn | gs://cmip6/CMIP6/CMIP/NCAR/CESM2/historical/r8... | NaN | 20190311 |
64914 | CMIP | NCAR | CESM2 | historical | r7i1p1f1 | Amon | tas | gn | gs://cmip6/CMIP6/CMIP/NCAR/CESM2/historical/r7... | NaN | 20190311 |
64983 | CMIP | NCAR | CESM2 | historical | r9i1p1f1 | Amon | tas | gn | gs://cmip6/CMIP6/CMIP/NCAR/CESM2/historical/r9... | NaN | 20190311 |
66341 | CMIP | NCAR | CESM2 | historical | r10i1p1f1 | Amon | tas | gn | gs://cmip6/CMIP6/CMIP/NCAR/CESM2/historical/r1... | NaN | 20190313 |
200772 | CMIP | NCAR | CESM2 | historical | r11i1p1f1 | Amon | tas | gn | gs://cmip6/CMIP6/CMIP/NCAR/CESM2/historical/r1... | NaN | 20190514 |
385224 | CMIP | NCAR | CESM2-FV2 | historical | r1i1p1f1 | Amon | tas | gn | gs://cmip6/CMIP6/CMIP/NCAR/CESM2-FV2/historica... | NaN | 20191120 |
386297 | CMIP | NCAR | CESM2-WACCM-FV2 | historical | r1i1p1f1 | Amon | tas | gn | gs://cmip6/CMIP6/CMIP/NCAR/CESM2-WACCM-FV2/his... | NaN | 20191120 |
420771 | CMIP | NCAR | CESM2-WACCM-FV2 | historical | r3i1p1f1 | Amon | tas | gn | gs://cmip6/CMIP6/CMIP/NCAR/CESM2-WACCM-FV2/his... | NaN | 20200226 |
421251 | CMIP | NCAR | CESM2-WACCM-FV2 | historical | r2i1p1f1 | Amon | tas | gn | gs://cmip6/CMIP6/CMIP/NCAR/CESM2-WACCM-FV2/his... | NaN | 20200226 |
422013 | CMIP | NCAR | CESM2-FV2 | historical | r3i1p1f1 | Amon | tas | gn | gs://cmip6/CMIP6/CMIP/NCAR/CESM2-FV2/historica... | NaN | 20200226 |
422459 | CMIP | NCAR | CESM2-FV2 | historical | r2i1p1f1 | Amon | tas | gn | gs://cmip6/CMIP6/CMIP/NCAR/CESM2-FV2/historica... | NaN | 20200226 |
Load Data
Now we will load a single store using fsspec
, zarr
, and xarray
.
# get the path to a specific zarr store (the first one from the dataframe above)
zstore = df_ta_ncar.zstore.values[-1]
print(zstore)
# create a mutable-mapping-style interface to the store
mapper = fsspec.get_mapper(zstore)
# open it using xarray and zarr
ds = xr.open_zarr(mapper, consolidated=True)
ds
gs://cmip6/CMIP6/CMIP/NCAR/CESM2-FV2/historical/r2i1p1f1/Amon/tas/gn/v20200226/
<xarray.Dataset> Size: 110MB Dimensions: (lat: 96, nbnd: 2, lon: 144, time: 1980) Coordinates: * lat (lat) float64 768B -90.0 -88.11 -86.21 ... 86.21 88.11 90.0 lat_bnds (lat, nbnd) float64 2kB dask.array<chunksize=(96, 2), meta=np.ndarray> * lon (lon) float64 1kB 0.0 2.5 5.0 7.5 ... 350.0 352.5 355.0 357.5 lon_bnds (lon, nbnd) float64 2kB dask.array<chunksize=(144, 2), meta=np.ndarray> * time (time) object 16kB 1850-01-15 12:00:00 ... 2014-12-15 12:00:00 time_bnds (time, nbnd) object 32kB dask.array<chunksize=(1980, 2), meta=np.ndarray> Dimensions without coordinates: nbnd Data variables: tas (time, lat, lon) float32 109MB dask.array<chunksize=(990, 96, 144), meta=np.ndarray> Attributes: (12/48) Conventions: CF-1.7 CMIP-6.2 DODS_EXTRA.Unlimited_Dimension: time activity_id: CMIP branch_method: standard branch_time_in_child: 674885.0 branch_time_in_parent: 10950.0 ... ... tracking_id: hdl:21.14100/99cdfde8-5b6d-452b-9b78-62a... variable_id: tas variant_info: CMIP6 CESM2-FV2 historical experiment (1... variant_label: r2i1p1f1 netcdf_tracking_ids: hdl:21.14100/99cdfde8-5b6d-452b-9b78-62a... version_id: v20200226
Plot the Data
Plot a map from a specific date:
ds.tas.sel(time='1950-01').squeeze().plot()
<matplotlib.collections.QuadMesh at 0x7fdb4019ad70>
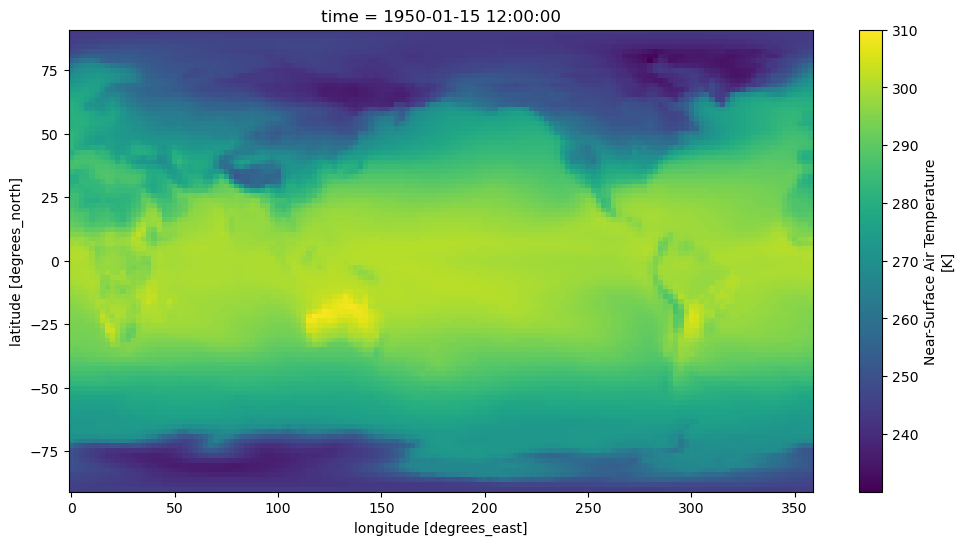
The global mean of a lat-lon field needs to be weighted by the area of each grid cell, which is proportional to the cosine of its latitude.
def global_mean(field):
weights = np.cos(np.deg2rad(field.lat))
return field.weighted(weights).mean(dim=['lat', 'lon'])
We can pass all of the temperature data through this function:
ta_timeseries = global_mean(ds.tas)
ta_timeseries
<xarray.DataArray 'tas' (time: 1980)> Size: 16kB dask.array<truediv, shape=(1980,), dtype=float64, chunksize=(990,), chunktype=numpy.ndarray> Coordinates: * time (time) object 16kB 1850-01-15 12:00:00 ... 2014-12-15 12:00:00
By default the data are loaded lazily, as Dask arrays. Here we trigger computation explicitly.
%time ta_timeseries.load()
CPU times: user 268 ms, sys: 95.7 ms, total: 364 ms
Wall time: 643 ms
<xarray.DataArray 'tas' (time: 1980)> Size: 16kB array([285.53603312, 285.63958225, 286.27324086, ..., 288.15781771, 287.18662389, 286.87765827]) Coordinates: * time (time) object 16kB 1850-01-15 12:00:00 ... 2014-12-15 12:00:00
ta_timeseries.plot(label='monthly')
ta_timeseries.rolling(time=12).mean().plot(label='12 month rolling mean', color='k')
plt.legend()
plt.grid()
plt.title('Global Mean Surface Air Temperature')
Text(0.5, 1.0, 'Global Mean Surface Air Temperature')
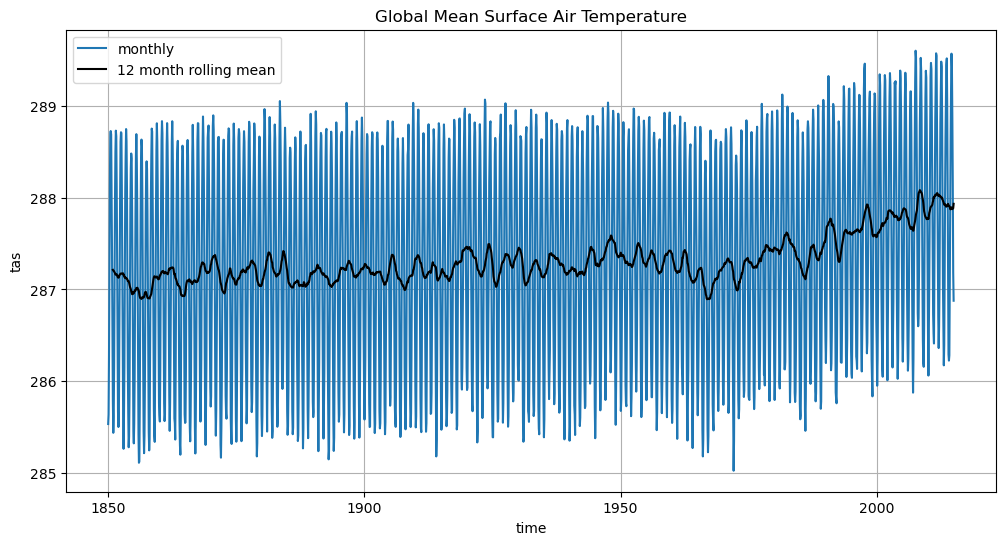
Summary
In this notebook, we opened a CESM2 dataset with fsspec
and zarr
. We calculated and plotted global average surface air temperature.
What’s next?
We will open a dataset with ESGF and OPenDAP.
Resources and references
Original notebook in the Pangeo Gallery by Henri Drake and Ryan Abernathey