Ocean iron concentration
Overview
Iron is one of the most important micronutrients in the ocean, limiting production in some areas. In this notebook, we make a map of iron concentration and compare it to observational data.
General setup
Subsetting
Processing - long-term mean
Comparing to observational data
Prerequisites
Concepts |
Importance |
Notes |
---|---|---|
Necessary |
||
Necessary |
||
Helpful |
||
Helpful |
Time to learn: 15 min
Imports
import xarray as xr
import glob
import numpy as np
import matplotlib.pyplot as plt
import cartopy
import cartopy.crs as ccrs
import pop_tools
from dask.distributed import LocalCluster
import pandas as pd
import s3fs
import netCDF4
from module import adjust_pop_grid
General setup (see intro notebooks for explanations)
Connect to cluster
cluster = LocalCluster()
client = cluster.get_client()
Bring in POP grid utilities
ds_grid = pop_tools.get_grid('POP_gx1v7')
lons = ds_grid.TLONG
lats = ds_grid.TLAT
depths = ds_grid.z_t * 0.01
Load the data
jetstream_url = 'https://js2.jetstream-cloud.org:8001/'
s3 = s3fs.S3FileSystem(anon=True, client_kwargs=dict(endpoint_url=jetstream_url))
# Generate a list of all files in CESM folder
s3path = 's3://pythia/ocean-bgc/cesm/g.e22.GOMIPECOIAF_JRA-1p4-2018.TL319_g17.4p2z.002branch/ocn/proc/tseries/month_1/*'
remote_files = s3.glob(s3path)
# Open all files from folder
fileset = [s3.open(file) for file in remote_files]
# Open with xarray
ds = xr.open_mfdataset(fileset, data_vars="minimal", coords='minimal', compat="override", parallel=True,
drop_variables=["transport_components", "transport_regions", 'moc_components'], decode_times=True)
ds
<xarray.Dataset> Size: 28GB Dimensions: (nlat: 384, nlon: 320, time: 120, z_t: 60, z_t_150m: 15) Coordinates: TLAT (nlat, nlon) float64 983kB dask.array<chunksize=(384, 320), meta=np.ndarray> TLONG (nlat, nlon) float64 983kB dask.array<chunksize=(384, 320), meta=np.ndarray> * time (time) object 960B 2010-01-16 12:00:00 ..... * z_t (z_t) float32 240B 500.0 ... 5.375e+05 * z_t_150m (z_t_150m) float32 60B 500.0 ... 1.45e+04 Dimensions without coordinates: nlat, nlon Data variables: (12/45) FG_CO2 (time, nlat, nlon) float32 59MB dask.array<chunksize=(60, 192, 160), meta=np.ndarray> Fe (time, z_t, nlat, nlon) float32 4GB dask.array<chunksize=(30, 15, 96, 80), meta=np.ndarray> NO3 (time, z_t, nlat, nlon) float32 4GB dask.array<chunksize=(30, 15, 96, 80), meta=np.ndarray> PO4 (time, z_t, nlat, nlon) float32 4GB dask.array<chunksize=(30, 15, 96, 80), meta=np.ndarray> POC_FLUX_100m (time, nlat, nlon) float32 59MB dask.array<chunksize=(60, 192, 160), meta=np.ndarray> SALT (time, z_t, nlat, nlon) float32 4GB dask.array<chunksize=(30, 15, 96, 80), meta=np.ndarray> ... ... sp_Fe_lim_Cweight_avg_100m (time, nlat, nlon) float32 59MB dask.array<chunksize=(60, 192, 160), meta=np.ndarray> sp_Fe_lim_surf (time, nlat, nlon) float32 59MB dask.array<chunksize=(60, 192, 160), meta=np.ndarray> sp_N_lim_Cweight_avg_100m (time, nlat, nlon) float32 59MB dask.array<chunksize=(60, 192, 160), meta=np.ndarray> sp_N_lim_surf (time, nlat, nlon) float32 59MB dask.array<chunksize=(60, 192, 160), meta=np.ndarray> sp_P_lim_Cweight_avg_100m (time, nlat, nlon) float32 59MB dask.array<chunksize=(60, 192, 160), meta=np.ndarray> sp_P_lim_surf (time, nlat, nlon) float32 59MB dask.array<chunksize=(60, 192, 160), meta=np.ndarray>
- nlat: 384
- nlon: 320
- time: 120
- z_t: 60
- z_t_150m: 15
- TLAT(nlat, nlon)float64dask.array<chunksize=(384, 320), meta=np.ndarray>
- long_name :
- array of t-grid latitudes
- units :
- degrees_north
Array Chunk Bytes 0.94 MiB 0.94 MiB Shape (384, 320) (384, 320) Dask graph 1 chunks in 2 graph layers Data type float64 numpy.ndarray - TLONG(nlat, nlon)float64dask.array<chunksize=(384, 320), meta=np.ndarray>
- long_name :
- array of t-grid longitudes
- units :
- degrees_east
Array Chunk Bytes 0.94 MiB 0.94 MiB Shape (384, 320) (384, 320) Dask graph 1 chunks in 2 graph layers Data type float64 numpy.ndarray - time(time)object2010-01-16 12:00:00 ... 2019-12-...
array([cftime.DatetimeNoLeap(2010, 1, 16, 12, 0, 0, 0, has_year_zero=True), cftime.DatetimeNoLeap(2010, 2, 15, 0, 0, 0, 0, has_year_zero=True), cftime.DatetimeNoLeap(2010, 3, 16, 12, 0, 0, 0, has_year_zero=True), cftime.DatetimeNoLeap(2010, 4, 16, 0, 0, 0, 0, has_year_zero=True), cftime.DatetimeNoLeap(2010, 5, 16, 12, 0, 0, 0, has_year_zero=True), cftime.DatetimeNoLeap(2010, 6, 16, 0, 0, 0, 0, has_year_zero=True), cftime.DatetimeNoLeap(2010, 7, 16, 12, 0, 0, 0, has_year_zero=True), cftime.DatetimeNoLeap(2010, 8, 16, 12, 0, 0, 0, has_year_zero=True), cftime.DatetimeNoLeap(2010, 9, 16, 0, 0, 0, 0, has_year_zero=True), cftime.DatetimeNoLeap(2010, 10, 16, 12, 0, 0, 0, has_year_zero=True), cftime.DatetimeNoLeap(2010, 11, 16, 0, 0, 0, 0, has_year_zero=True), cftime.DatetimeNoLeap(2010, 12, 16, 12, 0, 0, 0, has_year_zero=True), cftime.DatetimeNoLeap(2011, 1, 16, 12, 0, 0, 0, has_year_zero=True), cftime.DatetimeNoLeap(2011, 2, 15, 0, 0, 0, 0, has_year_zero=True), cftime.DatetimeNoLeap(2011, 3, 16, 12, 0, 0, 0, has_year_zero=True), cftime.DatetimeNoLeap(2011, 4, 16, 0, 0, 0, 0, has_year_zero=True), cftime.DatetimeNoLeap(2011, 5, 16, 12, 0, 0, 0, has_year_zero=True), cftime.DatetimeNoLeap(2011, 6, 16, 0, 0, 0, 0, has_year_zero=True), cftime.DatetimeNoLeap(2011, 7, 16, 12, 0, 0, 0, has_year_zero=True), cftime.DatetimeNoLeap(2011, 8, 16, 12, 0, 0, 0, has_year_zero=True), cftime.DatetimeNoLeap(2011, 9, 16, 0, 0, 0, 0, has_year_zero=True), cftime.DatetimeNoLeap(2011, 10, 16, 12, 0, 0, 0, has_year_zero=True), cftime.DatetimeNoLeap(2011, 11, 16, 0, 0, 0, 0, has_year_zero=True), cftime.DatetimeNoLeap(2011, 12, 16, 12, 0, 0, 0, has_year_zero=True), cftime.DatetimeNoLeap(2012, 1, 16, 12, 0, 0, 0, has_year_zero=True), cftime.DatetimeNoLeap(2012, 2, 15, 0, 0, 0, 0, has_year_zero=True), cftime.DatetimeNoLeap(2012, 3, 16, 12, 0, 0, 0, has_year_zero=True), cftime.DatetimeNoLeap(2012, 4, 16, 0, 0, 0, 0, has_year_zero=True), cftime.DatetimeNoLeap(2012, 5, 16, 12, 0, 0, 0, has_year_zero=True), cftime.DatetimeNoLeap(2012, 6, 16, 0, 0, 0, 0, has_year_zero=True), cftime.DatetimeNoLeap(2012, 7, 16, 12, 0, 0, 0, has_year_zero=True), cftime.DatetimeNoLeap(2012, 8, 16, 12, 0, 0, 0, has_year_zero=True), cftime.DatetimeNoLeap(2012, 9, 16, 0, 0, 0, 0, has_year_zero=True), cftime.DatetimeNoLeap(2012, 10, 16, 12, 0, 0, 0, has_year_zero=True), cftime.DatetimeNoLeap(2012, 11, 16, 0, 0, 0, 0, has_year_zero=True), cftime.DatetimeNoLeap(2012, 12, 16, 12, 0, 0, 0, has_year_zero=True), cftime.DatetimeNoLeap(2013, 1, 16, 12, 0, 0, 0, has_year_zero=True), cftime.DatetimeNoLeap(2013, 2, 15, 0, 0, 0, 0, has_year_zero=True), cftime.DatetimeNoLeap(2013, 3, 16, 12, 0, 0, 0, has_year_zero=True), cftime.DatetimeNoLeap(2013, 4, 16, 0, 0, 0, 0, has_year_zero=True), cftime.DatetimeNoLeap(2013, 5, 16, 12, 0, 0, 0, has_year_zero=True), cftime.DatetimeNoLeap(2013, 6, 16, 0, 0, 0, 0, has_year_zero=True), cftime.DatetimeNoLeap(2013, 7, 16, 12, 0, 0, 0, has_year_zero=True), cftime.DatetimeNoLeap(2013, 8, 16, 12, 0, 0, 0, has_year_zero=True), cftime.DatetimeNoLeap(2013, 9, 16, 0, 0, 0, 0, has_year_zero=True), cftime.DatetimeNoLeap(2013, 10, 16, 12, 0, 0, 0, has_year_zero=True), cftime.DatetimeNoLeap(2013, 11, 16, 0, 0, 0, 0, has_year_zero=True), cftime.DatetimeNoLeap(2013, 12, 16, 12, 0, 0, 0, has_year_zero=True), cftime.DatetimeNoLeap(2014, 1, 16, 12, 0, 0, 0, has_year_zero=True), cftime.DatetimeNoLeap(2014, 2, 15, 0, 0, 0, 0, has_year_zero=True), cftime.DatetimeNoLeap(2014, 3, 16, 12, 0, 0, 0, has_year_zero=True), cftime.DatetimeNoLeap(2014, 4, 16, 0, 0, 0, 0, has_year_zero=True), cftime.DatetimeNoLeap(2014, 5, 16, 12, 0, 0, 0, has_year_zero=True), cftime.DatetimeNoLeap(2014, 6, 16, 0, 0, 0, 0, has_year_zero=True), cftime.DatetimeNoLeap(2014, 7, 16, 12, 0, 0, 0, has_year_zero=True), cftime.DatetimeNoLeap(2014, 8, 16, 12, 0, 0, 0, has_year_zero=True), cftime.DatetimeNoLeap(2014, 9, 16, 0, 0, 0, 0, has_year_zero=True), cftime.DatetimeNoLeap(2014, 10, 16, 12, 0, 0, 0, has_year_zero=True), cftime.DatetimeNoLeap(2014, 11, 16, 0, 0, 0, 0, has_year_zero=True), cftime.DatetimeNoLeap(2014, 12, 16, 12, 0, 0, 0, has_year_zero=True), cftime.DatetimeNoLeap(2015, 1, 16, 12, 0, 0, 0, has_year_zero=True), cftime.DatetimeNoLeap(2015, 2, 15, 0, 0, 0, 0, has_year_zero=True), cftime.DatetimeNoLeap(2015, 3, 16, 12, 0, 0, 0, has_year_zero=True), cftime.DatetimeNoLeap(2015, 4, 16, 0, 0, 0, 0, has_year_zero=True), cftime.DatetimeNoLeap(2015, 5, 16, 12, 0, 0, 0, has_year_zero=True), cftime.DatetimeNoLeap(2015, 6, 16, 0, 0, 0, 0, has_year_zero=True), cftime.DatetimeNoLeap(2015, 7, 16, 12, 0, 0, 0, has_year_zero=True), cftime.DatetimeNoLeap(2015, 8, 16, 12, 0, 0, 0, has_year_zero=True), cftime.DatetimeNoLeap(2015, 9, 16, 0, 0, 0, 0, has_year_zero=True), cftime.DatetimeNoLeap(2015, 10, 16, 12, 0, 0, 0, has_year_zero=True), cftime.DatetimeNoLeap(2015, 11, 16, 0, 0, 0, 0, has_year_zero=True), cftime.DatetimeNoLeap(2015, 12, 16, 12, 0, 0, 0, has_year_zero=True), cftime.DatetimeNoLeap(2016, 1, 16, 12, 0, 0, 0, has_year_zero=True), cftime.DatetimeNoLeap(2016, 2, 15, 0, 0, 0, 0, has_year_zero=True), cftime.DatetimeNoLeap(2016, 3, 16, 12, 0, 0, 0, has_year_zero=True), cftime.DatetimeNoLeap(2016, 4, 16, 0, 0, 0, 0, has_year_zero=True), cftime.DatetimeNoLeap(2016, 5, 16, 12, 0, 0, 0, has_year_zero=True), cftime.DatetimeNoLeap(2016, 6, 16, 0, 0, 0, 0, has_year_zero=True), cftime.DatetimeNoLeap(2016, 7, 16, 12, 0, 0, 0, has_year_zero=True), cftime.DatetimeNoLeap(2016, 8, 16, 12, 0, 0, 0, has_year_zero=True), cftime.DatetimeNoLeap(2016, 9, 16, 0, 0, 0, 0, has_year_zero=True), cftime.DatetimeNoLeap(2016, 10, 16, 12, 0, 0, 0, has_year_zero=True), cftime.DatetimeNoLeap(2016, 11, 16, 0, 0, 0, 0, has_year_zero=True), cftime.DatetimeNoLeap(2016, 12, 16, 12, 0, 0, 0, has_year_zero=True), cftime.DatetimeNoLeap(2017, 1, 16, 12, 0, 0, 0, has_year_zero=True), cftime.DatetimeNoLeap(2017, 2, 15, 0, 0, 0, 0, has_year_zero=True), cftime.DatetimeNoLeap(2017, 3, 16, 12, 0, 0, 0, has_year_zero=True), cftime.DatetimeNoLeap(2017, 4, 16, 0, 0, 0, 0, has_year_zero=True), cftime.DatetimeNoLeap(2017, 5, 16, 12, 0, 0, 0, has_year_zero=True), cftime.DatetimeNoLeap(2017, 6, 16, 0, 0, 0, 0, has_year_zero=True), cftime.DatetimeNoLeap(2017, 7, 16, 12, 0, 0, 0, has_year_zero=True), cftime.DatetimeNoLeap(2017, 8, 16, 12, 0, 0, 0, has_year_zero=True), cftime.DatetimeNoLeap(2017, 9, 16, 0, 0, 0, 0, has_year_zero=True), cftime.DatetimeNoLeap(2017, 10, 16, 12, 0, 0, 0, has_year_zero=True), cftime.DatetimeNoLeap(2017, 11, 16, 0, 0, 0, 0, has_year_zero=True), cftime.DatetimeNoLeap(2017, 12, 16, 12, 0, 0, 0, has_year_zero=True), cftime.DatetimeNoLeap(2018, 1, 16, 12, 0, 0, 0, has_year_zero=True), cftime.DatetimeNoLeap(2018, 2, 15, 0, 0, 0, 0, has_year_zero=True), cftime.DatetimeNoLeap(2018, 3, 16, 12, 0, 0, 0, has_year_zero=True), cftime.DatetimeNoLeap(2018, 4, 16, 0, 0, 0, 0, has_year_zero=True), cftime.DatetimeNoLeap(2018, 5, 16, 12, 0, 0, 0, has_year_zero=True), cftime.DatetimeNoLeap(2018, 6, 16, 0, 0, 0, 0, has_year_zero=True), cftime.DatetimeNoLeap(2018, 7, 16, 12, 0, 0, 0, has_year_zero=True), cftime.DatetimeNoLeap(2018, 8, 16, 12, 0, 0, 0, has_year_zero=True), cftime.DatetimeNoLeap(2018, 9, 16, 0, 0, 0, 0, has_year_zero=True), cftime.DatetimeNoLeap(2018, 10, 16, 12, 0, 0, 0, has_year_zero=True), cftime.DatetimeNoLeap(2018, 11, 16, 0, 0, 0, 0, has_year_zero=True), cftime.DatetimeNoLeap(2018, 12, 16, 12, 0, 0, 0, has_year_zero=True), cftime.DatetimeNoLeap(2019, 1, 16, 12, 0, 0, 0, has_year_zero=True), cftime.DatetimeNoLeap(2019, 2, 15, 0, 0, 0, 0, has_year_zero=True), cftime.DatetimeNoLeap(2019, 3, 16, 12, 0, 0, 0, has_year_zero=True), cftime.DatetimeNoLeap(2019, 4, 16, 0, 0, 0, 0, has_year_zero=True), cftime.DatetimeNoLeap(2019, 5, 16, 12, 0, 0, 0, has_year_zero=True), cftime.DatetimeNoLeap(2019, 6, 16, 0, 0, 0, 0, has_year_zero=True), cftime.DatetimeNoLeap(2019, 7, 16, 12, 0, 0, 0, has_year_zero=True), cftime.DatetimeNoLeap(2019, 8, 16, 12, 0, 0, 0, has_year_zero=True), cftime.DatetimeNoLeap(2019, 9, 16, 0, 0, 0, 0, has_year_zero=True), cftime.DatetimeNoLeap(2019, 10, 16, 12, 0, 0, 0, has_year_zero=True), cftime.DatetimeNoLeap(2019, 11, 16, 0, 0, 0, 0, has_year_zero=True), cftime.DatetimeNoLeap(2019, 12, 16, 12, 0, 0, 0, has_year_zero=True)], dtype=object)
- z_t(z_t)float32500.0 1.5e+03 ... 5.375e+05
- long_name :
- depth from surface to midpoint of layer
- units :
- centimeters
- positive :
- down
- valid_min :
- 500.0
- valid_max :
- 537500.0
array([5.000000e+02, 1.500000e+03, 2.500000e+03, 3.500000e+03, 4.500000e+03, 5.500000e+03, 6.500000e+03, 7.500000e+03, 8.500000e+03, 9.500000e+03, 1.050000e+04, 1.150000e+04, 1.250000e+04, 1.350000e+04, 1.450000e+04, 1.550000e+04, 1.650984e+04, 1.754790e+04, 1.862913e+04, 1.976603e+04, 2.097114e+04, 2.225783e+04, 2.364088e+04, 2.513702e+04, 2.676542e+04, 2.854837e+04, 3.051192e+04, 3.268680e+04, 3.510935e+04, 3.782276e+04, 4.087846e+04, 4.433777e+04, 4.827367e+04, 5.277280e+04, 5.793729e+04, 6.388626e+04, 7.075633e+04, 7.870025e+04, 8.788252e+04, 9.847059e+04, 1.106204e+05, 1.244567e+05, 1.400497e+05, 1.573946e+05, 1.764003e+05, 1.968944e+05, 2.186457e+05, 2.413972e+05, 2.649001e+05, 2.889385e+05, 3.133405e+05, 3.379793e+05, 3.627670e+05, 3.876452e+05, 4.125768e+05, 4.375392e+05, 4.625190e+05, 4.875083e+05, 5.125028e+05, 5.375000e+05], dtype=float32)
- z_t_150m(z_t_150m)float32500.0 1.5e+03 ... 1.35e+04 1.45e+04
- long_name :
- depth from surface to midpoint of layer
- units :
- centimeters
- positive :
- down
- valid_min :
- 500.0
- valid_max :
- 14500.0
array([ 500., 1500., 2500., 3500., 4500., 5500., 6500., 7500., 8500., 9500., 10500., 11500., 12500., 13500., 14500.], dtype=float32)
- FG_CO2(time, nlat, nlon)float32dask.array<chunksize=(60, 192, 160), meta=np.ndarray>
- long_name :
- DIC Surface Gas Flux
- units :
- mmol/m^3 cm/s
- grid_loc :
- 2110
- cell_methods :
- time: mean
Array Chunk Bytes 56.25 MiB 7.03 MiB Shape (120, 384, 320) (60, 192, 160) Dask graph 8 chunks in 2 graph layers Data type float32 numpy.ndarray - Fe(time, z_t, nlat, nlon)float32dask.array<chunksize=(30, 15, 96, 80), meta=np.ndarray>
- long_name :
- Dissolved Inorganic Iron
- units :
- mmol/m^3
- grid_loc :
- 3111
- cell_methods :
- time: mean
Array Chunk Bytes 3.30 GiB 13.18 MiB Shape (120, 60, 384, 320) (30, 15, 96, 80) Dask graph 256 chunks in 2 graph layers Data type float32 numpy.ndarray - NO3(time, z_t, nlat, nlon)float32dask.array<chunksize=(30, 15, 96, 80), meta=np.ndarray>
- long_name :
- Dissolved Inorganic Nitrate
- units :
- mmol/m^3
- grid_loc :
- 3111
- cell_methods :
- time: mean
Array Chunk Bytes 3.30 GiB 13.18 MiB Shape (120, 60, 384, 320) (30, 15, 96, 80) Dask graph 256 chunks in 2 graph layers Data type float32 numpy.ndarray - PO4(time, z_t, nlat, nlon)float32dask.array<chunksize=(30, 15, 96, 80), meta=np.ndarray>
- long_name :
- Dissolved Inorganic Phosphate
- units :
- mmol/m^3
- grid_loc :
- 3111
- cell_methods :
- time: mean
Array Chunk Bytes 3.30 GiB 13.18 MiB Shape (120, 60, 384, 320) (30, 15, 96, 80) Dask graph 256 chunks in 2 graph layers Data type float32 numpy.ndarray - POC_FLUX_100m(time, nlat, nlon)float32dask.array<chunksize=(60, 192, 160), meta=np.ndarray>
- long_name :
- POC Flux at 100m
- units :
- mmol/m^3 cm/s
- grid_loc :
- 2110
- cell_methods :
- time: mean
Array Chunk Bytes 56.25 MiB 7.03 MiB Shape (120, 384, 320) (60, 192, 160) Dask graph 8 chunks in 2 graph layers Data type float32 numpy.ndarray - SALT(time, z_t, nlat, nlon)float32dask.array<chunksize=(30, 15, 96, 80), meta=np.ndarray>
- long_name :
- Salinity
- units :
- gram/kilogram
- grid_loc :
- 3111
- cell_methods :
- time: mean
Array Chunk Bytes 3.30 GiB 13.18 MiB Shape (120, 60, 384, 320) (30, 15, 96, 80) Dask graph 256 chunks in 2 graph layers Data type float32 numpy.ndarray - SiO3(time, z_t, nlat, nlon)float32dask.array<chunksize=(30, 15, 96, 80), meta=np.ndarray>
- long_name :
- Dissolved Inorganic Silicate
- units :
- mmol/m^3
- grid_loc :
- 3111
- cell_methods :
- time: mean
Array Chunk Bytes 3.30 GiB 13.18 MiB Shape (120, 60, 384, 320) (30, 15, 96, 80) Dask graph 256 chunks in 2 graph layers Data type float32 numpy.ndarray - TEMP(time, z_t, nlat, nlon)float32dask.array<chunksize=(30, 15, 96, 80), meta=np.ndarray>
- long_name :
- Potential Temperature
- units :
- degC
- grid_loc :
- 3111
- cell_methods :
- time: mean
Array Chunk Bytes 3.30 GiB 13.18 MiB Shape (120, 60, 384, 320) (30, 15, 96, 80) Dask graph 256 chunks in 2 graph layers Data type float32 numpy.ndarray - coccoC(time, z_t_150m, nlat, nlon)float32dask.array<chunksize=(40, 5, 128, 107), meta=np.ndarray>
- long_name :
- Coccolithophores Carbon
- units :
- mmol/m^3
- grid_loc :
- 3114
- cell_methods :
- time: mean
Array Chunk Bytes 843.75 MiB 10.45 MiB Shape (120, 15, 384, 320) (40, 5, 128, 107) Dask graph 81 chunks in 2 graph layers Data type float32 numpy.ndarray - cocco_C_lim_Cweight_avg_100m(time, nlat, nlon)float32dask.array<chunksize=(60, 192, 160), meta=np.ndarray>
- long_name :
- Coccolithophores C Limitation, carbon biomass weighted average over 0-100m
- units :
- 1
- grid_loc :
- 2110
- cell_methods :
- time: mean
Array Chunk Bytes 56.25 MiB 7.03 MiB Shape (120, 384, 320) (60, 192, 160) Dask graph 8 chunks in 2 graph layers Data type float32 numpy.ndarray - cocco_C_lim_surf(time, nlat, nlon)float32dask.array<chunksize=(60, 192, 160), meta=np.ndarray>
- long_name :
- Coccolithophores C Limitation, Surface
- units :
- 1
- grid_loc :
- 2110
- cell_methods :
- time: mean
Array Chunk Bytes 56.25 MiB 7.03 MiB Shape (120, 384, 320) (60, 192, 160) Dask graph 8 chunks in 2 graph layers Data type float32 numpy.ndarray - cocco_Fe_lim_Cweight_avg_100m(time, nlat, nlon)float32dask.array<chunksize=(60, 192, 160), meta=np.ndarray>
- long_name :
- Coccolithophores Fe Limitation, carbon biomass weighted average over 0-100m
- units :
- 1
- grid_loc :
- 2110
- cell_methods :
- time: mean
Array Chunk Bytes 56.25 MiB 7.03 MiB Shape (120, 384, 320) (60, 192, 160) Dask graph 8 chunks in 2 graph layers Data type float32 numpy.ndarray - cocco_Fe_lim_surf(time, nlat, nlon)float32dask.array<chunksize=(60, 192, 160), meta=np.ndarray>
- long_name :
- Coccolithophores Fe Limitation, Surface
- units :
- 1
- grid_loc :
- 2110
- cell_methods :
- time: mean
Array Chunk Bytes 56.25 MiB 7.03 MiB Shape (120, 384, 320) (60, 192, 160) Dask graph 8 chunks in 2 graph layers Data type float32 numpy.ndarray - cocco_N_lim_Cweight_avg_100m(time, nlat, nlon)float32dask.array<chunksize=(60, 192, 160), meta=np.ndarray>
- long_name :
- Coccolithophores N Limitation, carbon biomass weighted average over 0-100m
- units :
- 1
- grid_loc :
- 2110
- cell_methods :
- time: mean
Array Chunk Bytes 56.25 MiB 7.03 MiB Shape (120, 384, 320) (60, 192, 160) Dask graph 8 chunks in 2 graph layers Data type float32 numpy.ndarray - cocco_N_lim_surf(time, nlat, nlon)float32dask.array<chunksize=(60, 192, 160), meta=np.ndarray>
- long_name :
- Coccolithophores N Limitation, Surface
- units :
- 1
- grid_loc :
- 2110
- cell_methods :
- time: mean
Array Chunk Bytes 56.25 MiB 7.03 MiB Shape (120, 384, 320) (60, 192, 160) Dask graph 8 chunks in 2 graph layers Data type float32 numpy.ndarray - cocco_P_lim_Cweight_avg_100m(time, nlat, nlon)float32dask.array<chunksize=(60, 192, 160), meta=np.ndarray>
- long_name :
- Coccolithophores P Limitation, carbon biomass weighted average over 0-100m
- units :
- 1
- grid_loc :
- 2110
- cell_methods :
- time: mean
Array Chunk Bytes 56.25 MiB 7.03 MiB Shape (120, 384, 320) (60, 192, 160) Dask graph 8 chunks in 2 graph layers Data type float32 numpy.ndarray - cocco_P_lim_surf(time, nlat, nlon)float32dask.array<chunksize=(60, 192, 160), meta=np.ndarray>
- long_name :
- Coccolithophores P Limitation, Surface
- units :
- 1
- grid_loc :
- 2110
- cell_methods :
- time: mean
Array Chunk Bytes 56.25 MiB 7.03 MiB Shape (120, 384, 320) (60, 192, 160) Dask graph 8 chunks in 2 graph layers Data type float32 numpy.ndarray - diatC(time, z_t_150m, nlat, nlon)float32dask.array<chunksize=(40, 5, 128, 107), meta=np.ndarray>
- long_name :
- Diatom Carbon
- units :
- mmol/m^3
- grid_loc :
- 3114
- cell_methods :
- time: mean
Array Chunk Bytes 843.75 MiB 10.45 MiB Shape (120, 15, 384, 320) (40, 5, 128, 107) Dask graph 81 chunks in 2 graph layers Data type float32 numpy.ndarray - diat_Fe_lim_Cweight_avg_100m(time, nlat, nlon)float32dask.array<chunksize=(60, 192, 160), meta=np.ndarray>
- long_name :
- Diatom Fe Limitation, carbon biomass weighted average over 0-100m
- units :
- 1
- grid_loc :
- 2110
- cell_methods :
- time: mean
Array Chunk Bytes 56.25 MiB 7.03 MiB Shape (120, 384, 320) (60, 192, 160) Dask graph 8 chunks in 2 graph layers Data type float32 numpy.ndarray - diat_Fe_lim_surf(time, nlat, nlon)float32dask.array<chunksize=(60, 192, 160), meta=np.ndarray>
- long_name :
- Diatom Fe Limitation, Surface
- units :
- 1
- grid_loc :
- 2110
- cell_methods :
- time: mean
Array Chunk Bytes 56.25 MiB 7.03 MiB Shape (120, 384, 320) (60, 192, 160) Dask graph 8 chunks in 2 graph layers Data type float32 numpy.ndarray - diat_N_lim_Cweight_avg_100m(time, nlat, nlon)float32dask.array<chunksize=(60, 192, 160), meta=np.ndarray>
- long_name :
- Diatom N Limitation, carbon biomass weighted average over 0-100m
- units :
- 1
- grid_loc :
- 2110
- cell_methods :
- time: mean
Array Chunk Bytes 56.25 MiB 7.03 MiB Shape (120, 384, 320) (60, 192, 160) Dask graph 8 chunks in 2 graph layers Data type float32 numpy.ndarray - diat_N_lim_surf(time, nlat, nlon)float32dask.array<chunksize=(60, 192, 160), meta=np.ndarray>
- long_name :
- Diatom N Limitation, Surface
- units :
- 1
- grid_loc :
- 2110
- cell_methods :
- time: mean
Array Chunk Bytes 56.25 MiB 7.03 MiB Shape (120, 384, 320) (60, 192, 160) Dask graph 8 chunks in 2 graph layers Data type float32 numpy.ndarray - diat_P_lim_Cweight_avg_100m(time, nlat, nlon)float32dask.array<chunksize=(60, 192, 160), meta=np.ndarray>
- long_name :
- Diatom P Limitation, carbon biomass weighted average over 0-100m
- units :
- 1
- grid_loc :
- 2110
- cell_methods :
- time: mean
Array Chunk Bytes 56.25 MiB 7.03 MiB Shape (120, 384, 320) (60, 192, 160) Dask graph 8 chunks in 2 graph layers Data type float32 numpy.ndarray - diat_P_lim_surf(time, nlat, nlon)float32dask.array<chunksize=(60, 192, 160), meta=np.ndarray>
- long_name :
- Diatom P Limitation, Surface
- units :
- 1
- grid_loc :
- 2110
- cell_methods :
- time: mean
Array Chunk Bytes 56.25 MiB 7.03 MiB Shape (120, 384, 320) (60, 192, 160) Dask graph 8 chunks in 2 graph layers Data type float32 numpy.ndarray - diat_SiO3_lim_Cweight_avg_100m(time, nlat, nlon)float32dask.array<chunksize=(60, 192, 160), meta=np.ndarray>
- long_name :
- Diatom SiO3 Limitation, carbon biomass weighted average over 0-100m
- units :
- 1
- grid_loc :
- 2110
- cell_methods :
- time: mean
Array Chunk Bytes 56.25 MiB 7.03 MiB Shape (120, 384, 320) (60, 192, 160) Dask graph 8 chunks in 2 graph layers Data type float32 numpy.ndarray - diat_SiO3_lim_surf(time, nlat, nlon)float32dask.array<chunksize=(60, 192, 160), meta=np.ndarray>
- long_name :
- Diatom SiO3 Limitation, Surface
- units :
- 1
- grid_loc :
- 2110
- cell_methods :
- time: mean
Array Chunk Bytes 56.25 MiB 7.03 MiB Shape (120, 384, 320) (60, 192, 160) Dask graph 8 chunks in 2 graph layers Data type float32 numpy.ndarray - diazC(time, z_t_150m, nlat, nlon)float32dask.array<chunksize=(40, 5, 128, 107), meta=np.ndarray>
- long_name :
- Diazotroph Carbon
- units :
- mmol/m^3
- grid_loc :
- 3114
- cell_methods :
- time: mean
Array Chunk Bytes 843.75 MiB 10.45 MiB Shape (120, 15, 384, 320) (40, 5, 128, 107) Dask graph 81 chunks in 2 graph layers Data type float32 numpy.ndarray - diaz_Fe_lim_Cweight_avg_100m(time, nlat, nlon)float32dask.array<chunksize=(60, 192, 160), meta=np.ndarray>
- long_name :
- Diazotroph Fe Limitation, carbon biomass weighted average over 0-100m
- units :
- 1
- grid_loc :
- 2110
- cell_methods :
- time: mean
Array Chunk Bytes 56.25 MiB 7.03 MiB Shape (120, 384, 320) (60, 192, 160) Dask graph 8 chunks in 2 graph layers Data type float32 numpy.ndarray - diaz_Fe_lim_surf(time, nlat, nlon)float32dask.array<chunksize=(60, 192, 160), meta=np.ndarray>
- long_name :
- Diazotroph Fe Limitation, Surface
- units :
- 1
- grid_loc :
- 2110
- cell_methods :
- time: mean
Array Chunk Bytes 56.25 MiB 7.03 MiB Shape (120, 384, 320) (60, 192, 160) Dask graph 8 chunks in 2 graph layers Data type float32 numpy.ndarray - diaz_P_lim_Cweight_avg_100m(time, nlat, nlon)float32dask.array<chunksize=(60, 192, 160), meta=np.ndarray>
- long_name :
- Diazotroph P Limitation, carbon biomass weighted average over 0-100m
- units :
- 1
- grid_loc :
- 2110
- cell_methods :
- time: mean
Array Chunk Bytes 56.25 MiB 7.03 MiB Shape (120, 384, 320) (60, 192, 160) Dask graph 8 chunks in 2 graph layers Data type float32 numpy.ndarray - diaz_P_lim_surf(time, nlat, nlon)float32dask.array<chunksize=(60, 192, 160), meta=np.ndarray>
- long_name :
- Diazotroph P Limitation, Surface
- units :
- 1
- grid_loc :
- 2110
- cell_methods :
- time: mean
Array Chunk Bytes 56.25 MiB 7.03 MiB Shape (120, 384, 320) (60, 192, 160) Dask graph 8 chunks in 2 graph layers Data type float32 numpy.ndarray - mesozooC(time, z_t_150m, nlat, nlon)float32dask.array<chunksize=(40, 5, 128, 107), meta=np.ndarray>
- long_name :
- Mesozooplankton Carbon
- units :
- mmol/m^3
- grid_loc :
- 3114
- cell_methods :
- time: mean
Array Chunk Bytes 843.75 MiB 10.45 MiB Shape (120, 15, 384, 320) (40, 5, 128, 107) Dask graph 81 chunks in 2 graph layers Data type float32 numpy.ndarray - microzooC(time, z_t_150m, nlat, nlon)float32dask.array<chunksize=(40, 5, 128, 107), meta=np.ndarray>
- long_name :
- Microzooplankton Carbon
- units :
- mmol/m^3
- grid_loc :
- 3114
- cell_methods :
- time: mean
Array Chunk Bytes 843.75 MiB 10.45 MiB Shape (120, 15, 384, 320) (40, 5, 128, 107) Dask graph 81 chunks in 2 graph layers Data type float32 numpy.ndarray - photoC_TOT_zint(time, nlat, nlon)float32dask.array<chunksize=(120, 384, 320), meta=np.ndarray>
- long_name :
- Total C Fixation Vertical Integral
- units :
- mmol/m^3 cm/s
- grid_loc :
- 2110
- cell_methods :
- time: mean
Array Chunk Bytes 56.25 MiB 56.25 MiB Shape (120, 384, 320) (120, 384, 320) Dask graph 1 chunks in 2 graph layers Data type float32 numpy.ndarray - photoC_cocco_zint(time, nlat, nlon)float32dask.array<chunksize=(60, 192, 160), meta=np.ndarray>
- long_name :
- Coccolithophores C Fixation Vertical Integral
- units :
- mmol/m^3 cm/s
- grid_loc :
- 2110
- cell_methods :
- time: mean
Array Chunk Bytes 56.25 MiB 7.03 MiB Shape (120, 384, 320) (60, 192, 160) Dask graph 8 chunks in 2 graph layers Data type float32 numpy.ndarray - photoC_diat_zint(time, nlat, nlon)float32dask.array<chunksize=(60, 192, 160), meta=np.ndarray>
- long_name :
- Diatom C Fixation Vertical Integral
- units :
- mmol/m^3 cm/s
- grid_loc :
- 2110
- cell_methods :
- time: mean
Array Chunk Bytes 56.25 MiB 7.03 MiB Shape (120, 384, 320) (60, 192, 160) Dask graph 8 chunks in 2 graph layers Data type float32 numpy.ndarray - photoC_diaz_zint(time, nlat, nlon)float32dask.array<chunksize=(60, 192, 160), meta=np.ndarray>
- long_name :
- Diazotroph C Fixation Vertical Integral
- units :
- mmol/m^3 cm/s
- grid_loc :
- 2110
- cell_methods :
- time: mean
Array Chunk Bytes 56.25 MiB 7.03 MiB Shape (120, 384, 320) (60, 192, 160) Dask graph 8 chunks in 2 graph layers Data type float32 numpy.ndarray - photoC_sp_zint(time, nlat, nlon)float32dask.array<chunksize=(60, 192, 160), meta=np.ndarray>
- long_name :
- Small Phyto C Fixation Vertical Integral
- units :
- mmol/m^3 cm/s
- grid_loc :
- 2110
- cell_methods :
- time: mean
Array Chunk Bytes 56.25 MiB 7.03 MiB Shape (120, 384, 320) (60, 192, 160) Dask graph 8 chunks in 2 graph layers Data type float32 numpy.ndarray - spC(time, z_t_150m, nlat, nlon)float32dask.array<chunksize=(40, 5, 128, 107), meta=np.ndarray>
- long_name :
- Small Phyto Carbon
- units :
- mmol/m^3
- grid_loc :
- 3114
- cell_methods :
- time: mean
Array Chunk Bytes 843.75 MiB 10.45 MiB Shape (120, 15, 384, 320) (40, 5, 128, 107) Dask graph 81 chunks in 2 graph layers Data type float32 numpy.ndarray - sp_Fe_lim_Cweight_avg_100m(time, nlat, nlon)float32dask.array<chunksize=(60, 192, 160), meta=np.ndarray>
- long_name :
- Small Phyto Fe Limitation, carbon biomass weighted average over 0-100m
- units :
- 1
- grid_loc :
- 2110
- cell_methods :
- time: mean
Array Chunk Bytes 56.25 MiB 7.03 MiB Shape (120, 384, 320) (60, 192, 160) Dask graph 8 chunks in 2 graph layers Data type float32 numpy.ndarray - sp_Fe_lim_surf(time, nlat, nlon)float32dask.array<chunksize=(60, 192, 160), meta=np.ndarray>
- long_name :
- Small Phyto Fe Limitation, Surface
- units :
- 1
- grid_loc :
- 2110
- cell_methods :
- time: mean
Array Chunk Bytes 56.25 MiB 7.03 MiB Shape (120, 384, 320) (60, 192, 160) Dask graph 8 chunks in 2 graph layers Data type float32 numpy.ndarray - sp_N_lim_Cweight_avg_100m(time, nlat, nlon)float32dask.array<chunksize=(60, 192, 160), meta=np.ndarray>
- long_name :
- Small Phyto N Limitation, carbon biomass weighted average over 0-100m
- units :
- 1
- grid_loc :
- 2110
- cell_methods :
- time: mean
Array Chunk Bytes 56.25 MiB 7.03 MiB Shape (120, 384, 320) (60, 192, 160) Dask graph 8 chunks in 2 graph layers Data type float32 numpy.ndarray - sp_N_lim_surf(time, nlat, nlon)float32dask.array<chunksize=(60, 192, 160), meta=np.ndarray>
- long_name :
- Small Phyto N Limitation, Surface
- units :
- 1
- grid_loc :
- 2110
- cell_methods :
- time: mean
Array Chunk Bytes 56.25 MiB 7.03 MiB Shape (120, 384, 320) (60, 192, 160) Dask graph 8 chunks in 2 graph layers Data type float32 numpy.ndarray - sp_P_lim_Cweight_avg_100m(time, nlat, nlon)float32dask.array<chunksize=(60, 192, 160), meta=np.ndarray>
- long_name :
- Small Phyto P Limitation, carbon biomass weighted average over 0-100m
- units :
- 1
- grid_loc :
- 2110
- cell_methods :
- time: mean
Array Chunk Bytes 56.25 MiB 7.03 MiB Shape (120, 384, 320) (60, 192, 160) Dask graph 8 chunks in 2 graph layers Data type float32 numpy.ndarray - sp_P_lim_surf(time, nlat, nlon)float32dask.array<chunksize=(60, 192, 160), meta=np.ndarray>
- long_name :
- Small Phyto P Limitation, Surface
- units :
- 1
- grid_loc :
- 2110
- cell_methods :
- time: mean
Array Chunk Bytes 56.25 MiB 7.03 MiB Shape (120, 384, 320) (60, 192, 160) Dask graph 8 chunks in 2 graph layers Data type float32 numpy.ndarray
- timePandasIndex
PandasIndex(CFTimeIndex([2010-01-16 12:00:00, 2010-02-15 00:00:00, 2010-03-16 12:00:00, 2010-04-16 00:00:00, 2010-05-16 12:00:00, 2010-06-16 00:00:00, 2010-07-16 12:00:00, 2010-08-16 12:00:00, 2010-09-16 00:00:00, 2010-10-16 12:00:00, ... 2019-03-16 12:00:00, 2019-04-16 00:00:00, 2019-05-16 12:00:00, 2019-06-16 00:00:00, 2019-07-16 12:00:00, 2019-08-16 12:00:00, 2019-09-16 00:00:00, 2019-10-16 12:00:00, 2019-11-16 00:00:00, 2019-12-16 12:00:00], dtype='object', length=120, calendar='noleap', freq=None))
- z_tPandasIndex
PandasIndex(Index([ 500.0, 1500.0, 2500.0, 3500.0, 4500.0, 5500.0, 6500.0, 7500.0, 8500.0, 9500.0, 10500.0, 11500.0, 12500.0, 13500.0, 14500.0, 15500.0, 16509.83984375, 17547.904296875, 18629.126953125, 19766.02734375, 20971.138671875, 22257.828125, 23640.8828125, 25137.015625, 26765.419921875, 28548.365234375, 30511.921875, 32686.798828125, 35109.34765625, 37822.76171875, 40878.46484375, 44337.76953125, 48273.671875, 52772.80078125, 57937.2890625, 63886.26171875, 70756.328125, 78700.25, 87882.5234375, 98470.5859375, 110620.421875, 124456.6875, 140049.71875, 157394.640625, 176400.328125, 196894.421875, 218645.65625, 241397.15625, 264900.125, 288938.46875, 313340.46875, 337979.34375, 362767.03125, 387645.1875, 412576.8125, 437539.25, 462519.03125, 487508.34375, 512502.8125, 537500.0], dtype='float32', name='z_t'))
- z_t_150mPandasIndex
PandasIndex(Index([ 500.0, 1500.0, 2500.0, 3500.0, 4500.0, 5500.0, 6500.0, 7500.0, 8500.0, 9500.0, 10500.0, 11500.0, 12500.0, 13500.0, 14500.0], dtype='float32', name='z_t_150m'))
Subsetting
variables =['Fe']
keep_vars=['z_t','z_t_150m','dz','time_bound', 'time','TAREA','TLAT','TLONG'] + variables
ds = ds.drop_vars([v for v in ds.variables if v not in keep_vars])
ds.Fe.isel(time=0,z_t=0).plot()
<matplotlib.collections.QuadMesh at 0x7f27bc0d92e0>
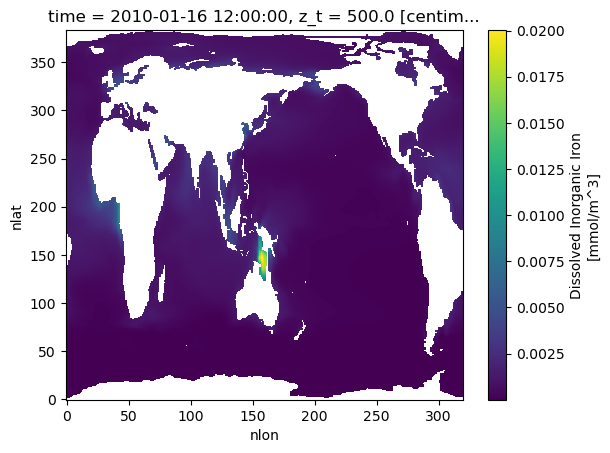
Processing - long-term mean
Pull in the function we defined in the nutrients notebook…
def year_mean(ds):
"""
Properly convert monthly data to annual means, taking into account month lengths.
Source: https://ncar.github.io/esds/posts/2021/yearly-averages-xarray/
"""
# Make a DataArray with the number of days in each month, size = len(time)
month_length = ds.time.dt.days_in_month
# Calculate the weights by grouping by 'time.year'
weights = (
month_length.groupby("time.year") / month_length.groupby("time.year").sum()
)
# Test that the sum of the year for each season is 1.0
np.testing.assert_allclose(weights.groupby("time.year").sum().values, np.ones((len(ds.groupby("time.year")), )))
# Calculate the weighted average
return (ds * weights).groupby("time.year").sum(dim="time")
Take the long-term mean of our data set. We process monthly to annual data with our custom function, then use xarray’s built-in .mean()
function to process from annual data to a single mean over time, since each year is the same length.
ds = year_mean(ds).mean("year")
nmolcm3_to_nM = 1.e3
ds['Fe'] = ds['Fe'] * nmolcm3_to_nM
ds['Fe'].attrs['units'] = 'nM'
Compare to observational Fe database
This dataset includes observations from the following papers:
as collected by Long et al., 2021 – see this paper for details.
fe_obs_path = 's3://pythia/ocean-bgc/obs/dFe-database-2021-05-20.csv'
fe_obs = s3.open(fe_obs_path)
df = pd.read_csv(fe_obs, na_values=-999.).dropna(axis=0, how='all')
df
lon | lat | depth | dFe_obs | |
---|---|---|---|---|
0 | 210.010 | -16.0018 | 20.0 | 0.540000 |
1 | 210.010 | -16.0018 | 35.0 | 0.440000 |
2 | 210.010 | -16.0019 | 50.0 | 0.480000 |
3 | 210.010 | -16.0019 | 80.0 | 0.400000 |
4 | 210.010 | -16.0020 | 100.0 | 0.390000 |
... | ... | ... | ... | ... |
27777 | 160.051 | 47.0032 | 3929.6 | 0.825681 |
27778 | 160.051 | 47.0032 | 3929.8 | 0.902248 |
27779 | 160.051 | 47.0032 | 4900.4 | 0.555630 |
27780 | 160.051 | 47.0032 | 4900.9 | 0.621851 |
27781 | 160.051 | 47.0032 | 5210.1 | 0.573220 |
27782 rows × 4 columns
fig = plt.figure(figsize=(16,5))
fig.suptitle("Surface iron concentration comparison")
### CESM
ax = fig.add_subplot(1,2,1, projection=ccrs.Robinson(central_longitude=305.0))
lon, lat, field = adjust_pop_grid(lons, lats, ds.Fe.isel(z_t=0))
pc=ax.pcolormesh(lon, lat, field, cmap='plasma',vmin=0,vmax=3,transform=ccrs.PlateCarree())
land = cartopy.feature.NaturalEarthFeature('physical', 'land', scale='110m', edgecolor='k', facecolor='white', linewidth=0.5)
ax.add_feature(land)
ax.set_title('CESM Fe at surface', fontsize=10)
### obs
ax = fig.add_subplot(1,2,2, projection=ccrs.Robinson(central_longitude=305.0))
ax.coastlines('10m',linewidth=0.5)
ax.set_title('Obs Fe at surface', fontsize=10)
df_sub = df.loc[(df.depth <= 2.5)]
sc = ax.scatter(df_sub.lon, df_sub.lat, c=df_sub.dFe_obs.values,
cmap='plasma',
vmin=0, vmax=3,
transform=ccrs.PlateCarree())
fig.subplots_adjust(right=0.8)
cbar_ax = fig.add_axes([0.85, 0.15, 0.02, 0.7])
fig.colorbar(pc, cax=cbar_ax, label='Fe (nM)')
<matplotlib.colorbar.Colorbar at 0x7f27ccc7eb40>
/home/runner/miniconda3/envs/ocean-bgc-cookbook-dev/lib/python3.12/site-packages/cartopy/io/__init__.py:241: DownloadWarning: Downloading: https://naturalearth.s3.amazonaws.com/10m_physical/ne_10m_coastline.zip
warnings.warn(f'Downloading: {url}', DownloadWarning)
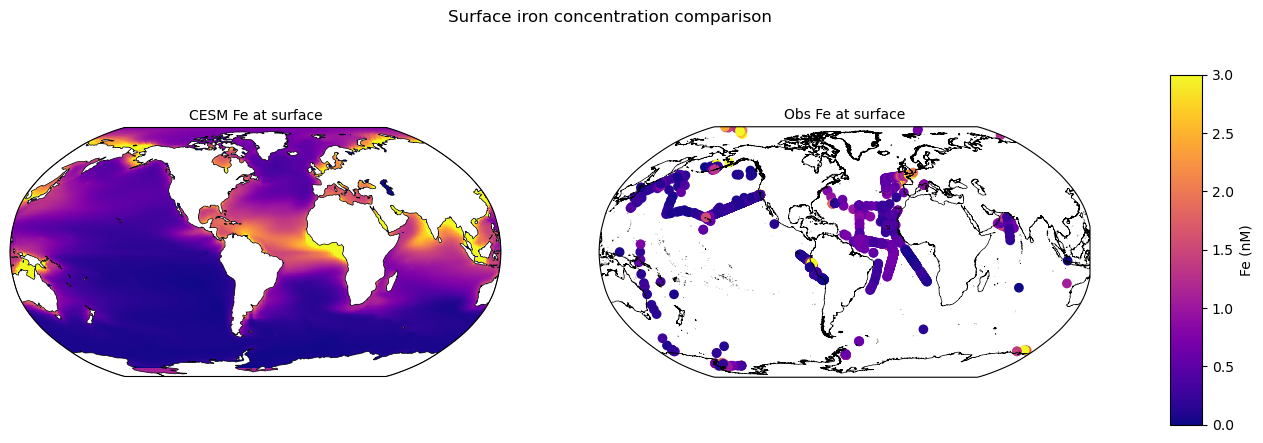
And close the Dask cluster we spun up at the beginning.
cluster.close()
Summary
You’ve learned how to make a map of ocean iron and compare it to observations.