Multi-file virtual datasets with VirtualiZarr
Overview
This notebook is intends to build off of the Basics of virtual Zarr stores.
In this tutorial we will:
Prerequisites
Concepts |
Importance |
Notes |
---|---|---|
Required |
Basic features |
|
Recommended |
IO |
Time to learn: 60 minutes
Flags
In the section below, set the subset
flag to be True
(default) or False
depending if you want this notebook to process the full file list. If set to True
, then a subset of the file list will be processed (Recommended)
subset_flag = True
Imports
In our imports block we are using similar imports to the Basics of virtual Zarr stores tutorial:
fsspec
for reading and writing to remote file systemsvirtualizarr
will be used to generate the virtual Zarr storeXarray
for examining the output dataset
import fsspec
import xarray as xr
from virtualizarr import open_virtual_dataset
Create a File Pattern from a list of input NetCDF files
Below we will create a list of input files we want to virtualize. In the Basics of virtual Zarr stores tutorial, we looked at a single file of climate downscaled data over Southern Alaska. In this example, we will build off of that work and use Kerchunk
and VirtualiZarr
to combine multiple NetCDF files of this dataset into a virtual dataset that can be read as if it were a Zarr
store - without copying any data.
We use the fsspec
s3
filesystem’s glob method to create a list of files matching a file pattern. We supply the base url of s3://wrf-se-ak-ar5/ccsm/rcp85/daily/2060/
, which is pointing to an AWS
public bucket, for daily rcp85 ccsm downscaled data for the year 2060. After this base url, we tacked on *
, which acts as a wildcard for all the files in the directory. We should expect 365 daily NetCDF
files.
Finally, we are appending the string s3://
to the list of return files. This will ensure the list of files we get back are s3
urls and can be read by VirtualiZarr
and Kerchunk
.
# Initiate fsspec filesystems for reading and writing
fs_read = fsspec.filesystem("s3", anon=True, skip_instance_cache=True)
# Retrieve list of available days in archive for the year 2060.
files_paths = fs_read.glob("s3://wrf-se-ak-ar5/ccsm/rcp85/daily/2060/*")
# Here we prepend the prefix 's3://', which points to AWS.
files_paths = sorted(["s3://" + f for f in files_paths])
As a quick check, it looks like we have a list 365 file paths, which should be a year of downscaled climte data.
print(f"{len(files_paths)} file paths were retrieved.")
365 file paths were retrieved.
# If the subset_flag == True (default), the list of input files will
# be subset to speed up the processing
if subset_flag:
files_paths = files_paths[0:4]
Optional: If you want to examine one NetCDF files before creating the Kerchunk
index, try uncommenting this code snippet below.
## Note: Optional piece of code to view one of the NetCDFs
# import s3fs
# fs = fsspec.filesystem("s3",anon=True)
# ds = xr.open_dataset(fs.open(file_pattern[0]))
Create virtual datasets for every file in the File_Pattern
list
Now that we have a list of NetCDF files, we can use VirtualiZarr
to create virtual datasets for each one of these.
Define kwargs for fsspec
In the cell below, we are creating a dictionary of kwargs
to pass to fsspec
and the s3
filesystem. Details on this can be found in the Basics of virtual Zarr stores tutorial in the Define storage_options arguments section
storage_options = dict(anon=True, default_fill_cache=False, default_cache_type="none")
In the cell below, we are reusing some of the functionality from the previous tutorial.
First we are defining a function named: generate_json_reference
.
This function:
Uses an
fsspec
s3
filesystem to read in aNetCDF
from a given url.Generates a
Kerchunk
index using theSingleHdf5ToZarr
Kerchunk
method.Creates a simplified filename using some string slicing.
Uses the local filesystem created with
fsspec
to write theKerchunk
index to a.json
reference file.
Below the generate_json_reference
function we created, we have a simple for
loop that iterates through our list of NetCDF
file urls and passes them to our generate_json_reference
function, which appends the name of each .json
reference file to a list named output_files.
virtual_datasets = [
open_virtual_dataset(
filepath, indexes={}, reader_options={"storage_options": storage_options}
)
for filepath in files_paths
]
Combine virtual datasets
After we have generated a virtual dataset for each NetCDF
file, we can combine these into a single virtual dataset using Xarray’s combine_nested
function.
combined_vds = xr.combine_nested(
virtual_datasets, concat_dim=["Time"], coords="minimal", compat="override"
)
combined_vds
<xarray.Dataset> Size: 123MB Dimensions: (Time: 4, soil_layers_stag: 4, south_north: 250, west_east: 320, interp_levels: 9) Coordinates: Time (Time) int32 16B ManifestArray<shape=(4,), dtype=int32, ch... interp_levels (interp_levels) float32 36B ManifestArray<shape=(9,), dtyp... Dimensions without coordinates: soil_layers_stag, south_north, west_east Data variables: (12/39) SH2O (Time, soil_layers_stag, south_north, west_east) float32 5MB ManifestArray<shape=(4, 4, 250, 320), dtype=float32, chunks=(1, 4, 250, 32... CLDFRA (Time, interp_levels, south_north, west_east) float32 12MB ManifestArray<shape=(4, 9, 250, 320), dtype=float32, chunks=(1, 9, 250, 320)... LWUPBC (Time, south_north, west_east) float32 1MB ManifestArray<s... LWUPB (Time, south_north, west_east) float32 1MB ManifestArray<s... PSFC (Time, south_north, west_east) float32 1MB ManifestArray<s... T (Time, interp_levels, south_north, west_east) float32 12MB ManifestArray<shape=(4, 9, 250, 320), dtype=float32, chunks=(1, 9, 250, 320)... ... ... SMOIS (Time, soil_layers_stag, south_north, west_east) float32 5MB ManifestArray<shape=(4, 4, 250, 320), dtype=float32, chunks=(1, 4, 250, 32... SWUPB (Time, south_north, west_east) float32 1MB ManifestArray<s... SNOW (Time, south_north, west_east) float32 1MB ManifestArray<s... lat (Time, south_north, west_east) float32 1MB ManifestArray<s... U10 (Time, south_north, west_east) float32 1MB ManifestArray<s... HFX (Time, south_north, west_east) float32 1MB ManifestArray<s...
Write combined virtual dataset to a Kerchunk JSON for future use
If we want to keep the combined reference information in memory as well as write the file to .json
, we can run the code snippet below.
# Write kerchunk .json record
output_fname = "combined_kerchunk.json"
combined_vds.virtualize.to_kerchunk(output_fname, format="json")
Using the output
Now that we have built a virtual dataset using VirtualiZarr
and Kerchunk
, we can read all of those original NetCDF
files as if they were a single Zarr
dataset.
**Since we saved the combined virtual dataset, this work doesn’t have to be repeated for anyone else to use this dataset. All they need is to pass the reference file storing the virtual dataset to Xarray
and it is as if they had a Zarr
dataset!
Open combined virtual dataset with Kerchunk
# We once again need to provide information for fsspec to access the remote file
storage_options = dict(
remote_protocol="s3", remote_options=dict(anon=True), skip_instance_cache=True
)
# We will use the "kerchunk" engine in `xr.open_dataset` and pass the `storage_options` to the `kerchunk` engine through `backend_kwargs`
ds = xr.open_dataset(
output_fname,
engine="kerchunk",
backend_kwargs={"storage_options": storage_options},
)
ds
<xarray.Dataset> Size: 123MB Dimensions: (Time: 4, south_north: 250, west_east: 320, interp_levels: 9, soil_layers_stag: 4) Coordinates: * Time (Time) float64 32B nan -2.147e+09 -2.147e+09 -2.147e+09 * interp_levels (interp_levels) float32 36B 100.0 200.0 300.0 ... 925.0 1e+03 Dimensions without coordinates: south_north, west_east, soil_layers_stag Data variables: (12/39) ACSNOW (Time, south_north, west_east) float32 1MB ... ALBEDO (Time, south_north, west_east) float32 1MB ... CLDFRA (Time, interp_levels, south_north, west_east) float32 12MB ... GHT (Time, interp_levels, south_north, west_east) float32 12MB ... HFX (Time, south_north, west_east) float32 1MB ... LH (Time, south_north, west_east) float32 1MB ... ... ... V (Time, interp_levels, south_north, west_east) float32 12MB ... V10 (Time, south_north, west_east) float32 1MB ... XLAT (Time, south_north, west_east) float32 1MB ... XLONG (Time, south_north, west_east) float32 1MB ... lat (Time, south_north, west_east) float32 1MB ... lon (Time, south_north, west_east) float32 1MB ...
Plot a slice of the dataset
Here we are using Xarray
to select a single time slice of the dataset and plot a map of snow cover over South East Alaska.
ds.isel(Time=0).SNOW.plot()
<matplotlib.collections.QuadMesh at 0x7fd0f308d240>
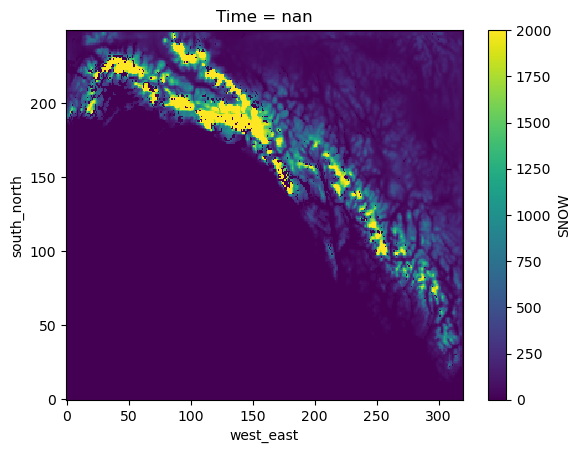